Plants in fire-prone ecosystems have evolved a variety of mechanisms to resist or adapt to fire. Post-fire resprouting is a key adaptation, promoting ecosystem recovery through rapid regeneration from underground or above-ground meristems after fire. Resprouting can have a significant impact on the terrestrial carbon cycle by promoting carbon sequestration after fires. The ability to resprout requires carbon investment to meristem formation and regrowth. Optimality theory would suggest that there must be a balance between the benefits of rapid recovery of photosynthesis against the costs of carbon storage and this implies that the incidence of resprouting will vary across biomes depending on the frequency and intensity of fires. We aim to develop a theoretical basis for including plant adaptations to fire as a function of costs versus benefits in different fire regimes, and the impacts of these adaptations on ecosystem dynamics, which will ultimately contribute to developing a more realistic model of the interactions between fire and ecosystems.
Three questions will be addressed in my project:
- Does community abundance of resprouting in woody plants reflect characteristics of the fire regime, such as fire return time, intensity, and type?
- Does the abundance of resprouters affect the time needed for ecosystem recovery after fire?
- Can the relationship between fire regimes and resprouting be modelled using optimality theory?
The first question has been answered in the paper we published in 2023. Please find the paper here if you are interested. Here are some briefs:
Our understanding of how the incidence of resprouting varies in different fire regimes is largely qualitative. The increasing availability of plant trait data and plot-based species cover data provides an opportunity to quantify the relationships between fire-related traits and fire properties. We investigated the quantitative relationship between fire frequency (expressed as the fire return time) and the proportion of resprouters in woody plants using plot data on species cover from Australia and Europe. We also examined the relationship between the proportion of resprouters and gross primary production (GPP) and grass cover, where GPP was assumed to reflect fuel loads and hence fire intensity, while grass cover was considered to be an indicator of the likelihood of ground fire and the speed of fire spread, using generalised linear modelling. We found that plants with the ability to resprout occur more often where fire regimes are characterised by high-frequency and high-intensity crown fires.
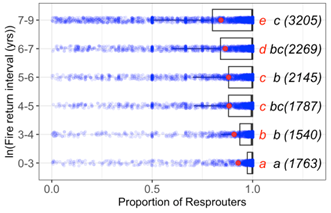
Figure 2 in Shen et al. 2023. Changes in the incidence of resprouting woody plants as a function of the fire return interval.
From that study, we know that resprouters are more abundant in regions with shorter fire return times, indicating that resprouting is an adaptation to frequent fires. We then want to know how quickly ecosystems recover after fire and what impact the abundance of resprouters has on recovery time because this could have a significant impact on the terrestrial carbon cycle by promoting substantial carbon sequestration. Currently, we are trying to quantify the relationship between the abundance of resprouters in an ecosystem and post-fire recovery time.